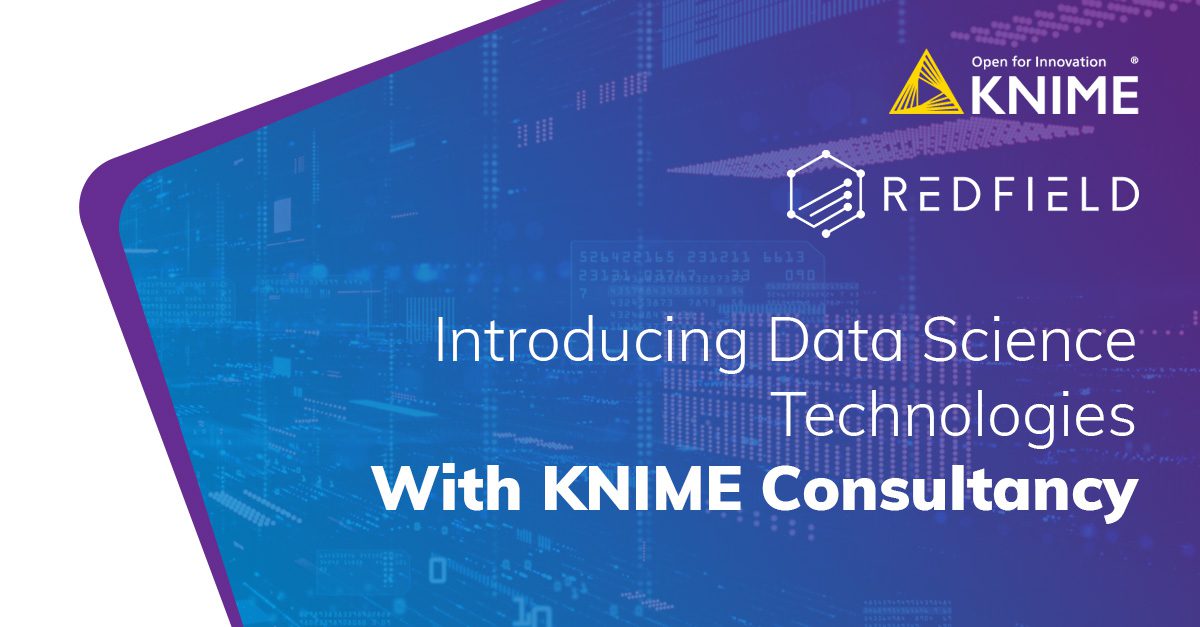
KNIME consultancy is an environment for sharing knowledge, collaborating, training, and launching data tools into production across organizations. This intuitive platform for understanding data and designing data science workflows enables companies to utilize big data to drive business strategies and become more confident, proactive, and financially savvy in the decision-making process.
Effectual change and improvement start and end with people. The human side of each problem-solving process ensures that the future of the enterprise relies on approaches, data science solutions, and expertise, with continuous enhancement of the workflows. Undoubtedly, many businesses lack the experience and bandwidth to implement change initiatives to extract the most value and avoid the commoditized feature of rival companies.
KNIME consulting experts are highly skilled at providing customized services in a way that interlocks the unique needs, budget, and work culture of each business. KNIME implementation ensures that company requirements and costs are observed from the client’s perspective. With the KNIME implementation and consultancy LLP services, enterprises receive elegant solutions using cutting-edge technologies that typically exceed all expectations.
Each project with the KNIME analytics platform, supported by KNIME consultancy and KNIME consultancy LLP team, is followed with professionalism and integrity. KNIME experts are adapting both the language and needs of the specific business and the language of the rapidly changing technology landscape while employing innovative approaches that aim to transform organizations and deliver sustainable business growth through business processes, strategies, and technology solutions.
Living in the era of new-age technology has brought about an explosion in data, resulting in the culmination of faster and better products and services. Consequently, the increase in value in data and the need to harness big and data driven decisions has tremendously risen over the last decade; hence, organizations employ methodologies that will center their business on data driven decisions.
Table of Contents
What Is Data Analytics?
Data analytics is a process in which the raw data is converted into meaningful, actionable insights. It includes a range of tools, technologies, and operations used to find trends hidden in data and improve the problem-solving process with facts backed by relevant information. Data analysis can shape business processes in enterprises, enhance decision-making with applicable insights, and foster business growth.
Examining data and valuable information hidden within big data helps organizations draw conclusions about the information they contain and the data they possess. Analysis of data is a multidisciplinary field that employs a wide range of analytics techniques, including math, statistics, and computer science. The process is progressively done with the aid of specialized systems and software that speed up the process, enhance it and bypass the possibility of human error when analyzing data. Various industries make more-informed business decisions thanks to the analysis of data, while scientists and researchers utilize analytics tools to verify or dispose of scientific models, hypotheses, and theories.
Data analytics has a broad focus and, as a term, predominately refers to an array of applications, ranging from basic business intelligence, reporting, and online analytical processing to various forms of advanced analytics. These initiatives help enterprises to increase revenue, improve operational efficiency and effectiveness, optimize marketing campaigns, and bolster customer service efforts. Additionally, by analyzing data, organizations promptly respond to emerging market trends and gain a competitive edge over business rivals.
The analysis of data can be performed over historical records or new information processed for real-time analytics, depending on the purposes and application. In the analysis process, companies can utilize data derived from a mix of internal systems and external data sources. Data analysts extract the raw data, organize it, and then analyze it, transforming it from incomprehensible characters into intelligible information.
How Is Data Analytics Used?
Data is everywhere. People use and produce data daily, whether they realize it or not. Daily tasks, such as checking the latest news or the daily weather report, tracking steps throughout the day, and searching for the latest fashion trends, are examples of data, or more precisely, forms of producing, analyzing, and using data.
At a high level, data analysis methodologies include exploratory data analysis (finding patterns and relationships in data) and confirmatory data analysis (statistical techniques to determine whether hypotheses about a data set are true or false). Data analytics can also be separated into quantitative data analysis (analysis of numerical data with quantifiable variables) and qualitative data analysis (understanding the content of non-numerical data like text, images, audio and video, common phrases, themes, and points of view). Additionally, at the application level, data analysis provides business executives and corporate workers with actionable information about key performance indicators, business operations, customers, and more.
There are advanced types of data analytics, including data mining (sorting through extensive data sets to identify trends, patterns, and relationships), predictive analytics (analyzing customer behavior, equipment failures, and other events and scenarios), machine learning (running automated algorithms to churn through data sets more quickly than manually by data scientists), text mining (analysis of documents and document classification, emails, and other text-based data), and more.
Inside the Data Analytics Process
Data analytics applications are much more than just analyzing data. Although, at first glance, analysis of data may seem to be a straightforward task, the truth is that processes, and workflows, particularly on advanced analytics projects (including contract analytics), are extensive and challenging. Much of the required work takes place upfront in the process of collecting data, integrating and preparing the data, followed by the workflows developing, testing, and revising analytical models to ensure that those processes produce accurate and relevant results.
The analytics process starts with data collection. Based on the particular analytics application, data scientists identify the information they need to extract from big data and work closely with data engineers and IT staff to assemble the data set. Data comes from different sources and in various formats, and to be suitable for analysis, the data needs to be combined through data integration processes, transformed into a standard format, and loaded into an analytics system, such as a data warehouse. Furthermore, after the data is collected and placed into the base, the next step is fixing data quality issues (through data profiling and data cleansing) that might affect the data accuracy. Once the data preparation process is finished, organizations must ensure that data governance policies are applied and that data follow corporate standards.
Using analytics software, predictive modeling tools, or programming languages enables data scientists to build the analytical model and initially run a test (or several tests) against a partial data set to check accuracy. Finally, the trained model is run in production mode against the full data set after the specific information is updated.
In some cases, the results of data analysis have an immediate and automated application and trigger business actions. For instance, if the data analysis shows that stock trades hit a specific price, a trigger can activate to buy more or sell all stocks without human involvement and approval. Otherwise, data analysis extracts results, and the information, in the form of reports or charts, is reviewed by business executives to help the decision-making process.
Data Analytics vs. Data Science
Automation grows daily, and, as a result, data scientists in the future will focus on business needs, deep learning, and strategic oversight. Data analysis will most likely concentrate on model creation and routine tasks. Generally, data scientists are dedicated to producing broad insights, while data analysis is focused on answering specific questions.
What Is Data Science?
Data science is a field that combines math and statistics expertise, specialized programming, advanced analytics, artificial intelligence (AI), and machine learning (ML) with specific subject matter expertise – to uncover actionable insights hidden in data and support data driven decisions. The acquisition, manipulation, visualization, analysis, and reporting performed with analysis tools, services and platforms guide decision-making and support strategic planning.
The accelerating volume of data sources, and consequently, the value of big data, has made data science the fastest-growing field implemented across every industry. Enterprises rely on data interpretation to provide actionable recommendations to customers, show costs, predict behaviors and improve business outcomes.
Data Science Use Cases
Data science is administered in businesses of all kinds, types, and sizes, from Fortune 50 companies to startups and small businesses. It is a rapidly growing field that revolutionizes many industries with complex data analysis, predictive modeling, data visualization, and recommendation engines.
Analysis of Complex Data
Data science provides quick and precise analysis of big data sets. With a variety of software tools and techniques available, data analysts identify trends and detect patterns within the largest and most complex data sets. This data science feature enables enterprises to make better decisions about the best-performing sector or conduct market analysis for new products.
Predictive Modeling
By analyzing big data sets, data science has the power to find and determine patterns in data by using machine learning. With a defined degree of accuracy, these models can forecast possible outcomes for customers, which is especially helpful in the marketing, insurance, and finance industries.
Recommendation Generation
Big companies like Netflix, Amazon, and Spotify rely on data science to generate user recommendations based on past behavior. This software helps enterprises and similar platforms gather content uniquely tailored to their preferences and interests.
Data Visualization
Graphs, charts, and dashboards are some of the most common methods for data visualization that help non-technical business leaders to analyze and understand complex information. Visual representation of the results makes the results easily readable so that anyone can understand the state of the enterprise.
Data Science Tools
Throughout their careers, data science professionals utilize an arsenal of data science tools and programming languages. The most popular options used today are:
Common Data Science Programming Languages
- Python
- SQL
- SAS
- R
- C/C++
- Java
- JavaScript
Popular Data Science Tools
- KNIME consultancy LLP (data analytics tool)
- RapidMiner
- Apache Spark (data analytics tool)
- Alteryx (analytics automation platform)
- Microsoft Excel (data analytics tool)
- Microsoft Power BI (business intelligence data analytics and data visualization tool)
- SAS (data analytics tool)
- Tableau (data visualization tool)
- TensorFlow (machine learning tool)
- Python
- MATLAB
End-To-End Data Science
In today’s digital world, experts in the field of data analysis advise and provide confidence to customers, boards, partners and stakeholders through every step of the transformation. Different aspects of analyzing data lead to delivering quality services that help enterprises grow, protect businesses, and build a better working world.
Some critical areas consultancy companies should cover are strategy, innovation, experience, operations, and trust. Our suggestion for a reliable and trustworthy data analysis tool is KNIME consultancy by KNIME consultancy LLP. With KNIME consultancy, enterprises get support in determining the purposes by unifying the digital approach while building end-to-end innovation to incubate new ideas and business models. Thanks to their extensive experience in digital technologies, KNIME experts continuously reinvent and implement better customer experiences while helping clients to understand data culture and how digital technology can help optimize processes and free resources.
KNIME Analytics Platform
KNIME Analytics Platform is open-source software that allows users to access, blend, analyze, and visualize data. This low-code, no-code development interface requires zero coding knowledge, gives support and offers an uncomplicated introduction for beginners while offering access to an advanced data science set of tools for experienced users.
Thanks to the intuitive KNIME services, drag-and-drop interface, users can create workflows by joining nodes together without the need for coding. KNIME analytics platform is a versatile software that offers automated spreadsheets, ETL (Extract, Transform, Load), predictive modeling, and machine learning. This way, through KNIME implementation, the software addresses data science needs of any complexity. Users can script in Phyton or R to further extend the capability.
KNIME consultancy LLP is one of the best examples of community-driven innovation. Thanks to KNIME’s open-source approach to development and support, users are on the cutting edge of data science with outstanding performance, integration to all popular machine learning libraries and over 300 connectors to data sources.
KNIME consultancy LLP breaks down barriers by blending different data types: strings, integers, images, text, networks, sound, molecules, and more. Furthermore, users can connect to all major databases and data warehouses, such as SQL Server, Postgres, MySQL, Snowflake, Redshift, BigQuery, and more.
What is even more important, KNIME consultancy LLP allows users to implement complex machine learning models without any programming knowledge necessary and shape, sort, filter, and join their data to derive statistics into different workflows and services.
Thanks to its simplicity, KNIME consulting empowers users to focus on the theory rather than the programmatic application. With KNIME consulting, AI, and deep learning are accessible. What makes KNIME consultancy services different from other vendors’ services is the divergency in enabling data analysis and data science development at the same time.
Additionally, you can check this video tutorial and learn more about KNIME:
FAQs
What Is KNIME Consultancy?
KNIME consultancy team and KNIME consultancy LLP offer an environment for sharing, collaborating, training and launching data tools into production across organizations. The intuitive platform of KNIME consultancy for understanding data and designing data science workflows enables companies to utilize big data to drive business strategies and become more confident, proactive, and financially savvy in the decision-making process.
What Is KNIME Analytics Platform?
KNIME Analytics Platform by KNIME consultancy LLP is open-source software that allows users to access, blend, analyze, and visualize data. This low-code, no-code interface requires zero coding and offers an uncomplicated introduction for beginners while offering an advanced data science set of tools for experienced users.
What Is Data Analytics?
Data analytics is a process in which the raw data is converted into meaningful, actionable insights. It includes a range of tools, technologies, and operations used to find trends hidden in data and improve the problem-solving process with facts backed by relevant information.