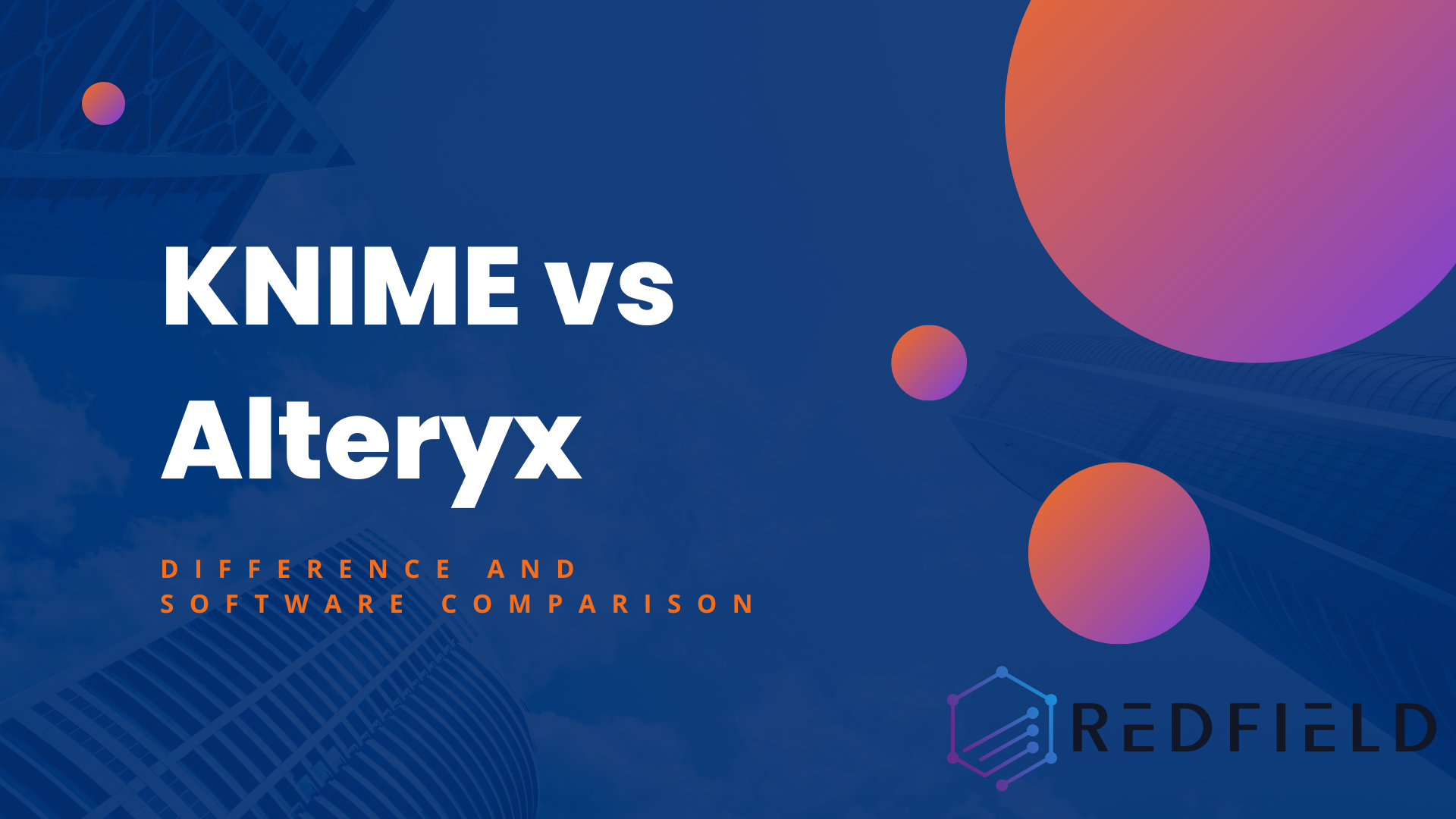
Having the best ETL tool will ease the various data science-related tasks and accordingly guide the decision-making and strategic planning. And this blog, KNIME vs Alteryx, is bound to be helpful for any business and organization. These top data science tools with ETL proficiency can help companies with the challenge of working with high data volumes, and this blog aims to compare the two potent tools in depth. Nonetheless, the user’s goal for data analytics purposes determines the best option.
One of the hallmarks of the Digital Age is that data exists everywhere. We access and use data to make informed decisions and to set our goals, which include searching for low-priced flights and hotels, review analytics of the average screen time, and estimated delivery dates of our packages. Organizations leverage data in the same way but on a larger scale.
Businesses embrace digital transformation and consolidate data about customers, employees, products, and services from multiple sources manipulating data mine, later used by business intelligence. Data science enables the centralized data repository to be filled with the collected data securely and organized so that the companies can gain timely data-driven insights.
Consequently, ETL tools and software have become indispensable for progressive and dynamic data analysts and organizations. The data-driven businesses transform the extracted data from different sources and load it into a database or data warehouse for reporting and analytics.
But what does ETL stand for? How do open-source software, computer software companies, data analytics, reporting, and integration platforms work?
Table of Contents
Introduction to ETL Tools
ETL is a term often used in data-related dialogs and refers to processes allowing businesses to access, modify, and store data.
What Is ETL?
ETL is a data integration process for the computation and analysis of data from multiple data sources into a single, consistent data store located in a data warehouse or other target system. ETL stands for extract, transform, and load and has become a primary method for various big data and science projects. It is a starting point for data analytics and machine learning workstreams and is used to enhance back-end processes or end-user experiences.
ETL tools are software designed to support the processes, including extracting data from legacy systems and different sources, scrubbing the collected data for uniformity and quality, and integrating the data into a target database. The standardized ETL process and tools approach simplifies data management strategies and improves data quality.
Types of ETL Tools
Based on the infrastructure and supporting organization, there are several ETL tools groups:
Enterprise Software ETL Tools
These software tools are developed and supported by commercial organizations and are some of the first ETL tools available in the marketplace. These tools offer great functionality through graphical user interfaces (GUIs) for architecting ETL pipelines, support for most relational and non-relational databases, and extensive documentation and user groups. The downside is the high price tag and employee training due to their complexity.
Open-Source ETL Tools
The open-source ETL tools entered the marketplace with the rise of the open-source movement. Most of these tools are free and offer GUIs for drafting data-sharing and monitoring the flow of information. The upper hand of open-source ETL solutions is that companies can access the source code, survey the tool’s infrastructure, and expand their capabilities. They can vary in documentation, maintenance, and functionality.
Cloud-Based ETL Tools
Organizations hugely accept cloud and integration platform service technologies, and thus, cloud service providers (CSPs) created ETL tools built on their infrastructure. The efficiency of the cloud-based ETL tools is one of the most significant advantages, which includes high latency, availability, and elasticity. This is exceptionally efficient when the organization uses the same CSP for data storage and all processes happen within the shared infrastructure. The only flaw is that these tools only work within CSP’s environment.
Custom ETL Tools
Businesses with resources for development often opt for proprietary ETL tools. By using programming languages (such as SQL, Python, and Java), companies use flexible ETL tools and take advantage of customized solutions for their business priorities. The most considerable drawback is the need for experts and internal resources for building a custom ETL tool and for the testing process, maintenance, and updates.
How to Evaluate ETL Tools
Every company has a unique business model and culture, and the collected data is used to enhance the company’s stability and success, including excellent or great customer experience, and the improvement and development of new models of products and services. Based on several criteria, companies can measure which ETL tool is relevant for their organization:
- Use case: the critical aspect for consideration for ETL tools is the size of the company and data analysis requirements. Namely, if the company is small and the analysis needed is minor, there is no need for a robust solution; conversely, large organizations require complex datasets.
- Budget: the assets at the company’s disposal for investing in ETL software are another crucial factor that has to be considered when evaluating the most suitable tool. Open-source tools are usually free but might not provide the support as enterprise-grade tools.
- Capabilities: ETL tools can be customized to meet the data need for business processes, while automated features enforce data quality and reduce the work required.
- Data sources: the most straightforward way to use data by ETL tools is the tool to have the ability to meet data at its original storage, whether it is on-premise or in the cloud. Organizations often have complex structured, and unstructured data structures in various formats; extracting information in a standardized format is ideal.
Why use ETL tools?
Organizations have relied on the ETL processes for many years to get an integrated data view. In fact, reporting and analytics are the main reasons why ETL tools exist. The structured and unstructured data from multiple systems and sources are formatted to be analyzed with data science to yield information for better business decisions.
As the data is loaded into the enterprise data warehouse (data at rest), the ETL tool sets the stage for long-term analysis and provides deep historical context for the business. The business intelligence tools then generate interactive and expedient reports for end-users and data visualization for managers and executives. Moreover, the consolidated view enables enterprises to analyze relevant data.
There are several different ways that ETL is most commonly used: data warehousing, cloud migration, machine learning, and AI and marketing data integration. Examples include banking data, insurance claims, and retail sales history; Internet of Things (IoT) devices pull loaded data for machine learning applications. The use cases are practically endless.
What is KNIME?
KNIME is a free-to-use, open-source data analytics, and reporting tool. This popular Business intelligence tool with modular data pipelining efficiently integrates multiple data mining and machine learning components. The data science software simplifies data, computerizes the processing of data science workflow, and enables accessibility of the reusable components to multiple users.
The KNIME services have a fully licensed tool that performs a wide range of data reporting and analytic operations and uses a graphical user interface (GUI) for more straightforward data analysis, better data visualization, an intuitive user interface, and improved overall user experience. KNIME is written in JAVA and Eclipse-based language.
KNIME Analytics Platform is a user-friendly software that enables users to access, merge, analyze, visualize, and transform data without coding. Through data apps and services, users can share new insights within the organization, including the potential hidden in the collected data, mine for fresh insights, or predict new features. Additionally, the no-code interface is accessible for use for beginners and, at the same time, provides an advanced data science set of tools for experienced users. Here is a visual presentation of how the platform looks like and which features are included:
This video tutorial offers a great introduction to the KNIME Analytics Platform for users of all levels: beginners, those who successfully navigate, and those somewhere in between.
What is Alteryx?
Alteryx is a data editing, analysis, and output software solution for organizations that need to handle large amounts of data. Users can prep, blend, analyze, and interpret data with easy end-to-end automation of data engineering, analytics, reporting, machine learning, and data science processes.
The Alteryx Analytics Automation Platform enables users to develop their paths independently, and the multiple tools blended into one platform enable data analysis and insights at the user community organization-wide level. Moreover, Alteryx has augmented machine learning which helps users speedily build predictive models without coding and is excellent for executing complex statistics.
The human-readable GUI enables users to monitor and operate with data, collaborate, and assist in the information flow. Scalability is another benefit for enterprises because there is an option for automated analysis across cloud, on-prem, and hybrid sources. Users with advanced knowledge can add Python and R code into data workflows and extract unstructured text data from PDF docs.
Head-to-head comparison: KNIME vs Alteryx
Briefly explained, KNIME is a data analysis, reporting, and integration tool that is free and open-source, and Alteryx is a software solution for data access, modification, analytics, and output. We will explain in detail about KNIME vs Alteryx tools when used for various data science purposes.
The same KNIME based modular data pipelining concept “Building Blocks of Analytics” enables integrated machine learning and data mining components. The GUI (graphical user interface), combined with JDBC (Java™ database connectivity), enables the creation of nodes that connect several different data sources for modeling, analysis, and visualization, all without or with very little code. The Alteryx processes allow users to observe, collaborate on, support, and improve. The software can read and write to files, databases, and APIs (Application Programming Interface). Additionally, Alteryx offers spatial analysis and predictive analytics and other tools too.
KNIME and Alteryx offer many common features regarding data science tools. However, the KNIME vs Alteryx differences make it easier for organizations to determine which solution suits their needs for specific use cases. For some, the customization and reliability provided by KNIME are crucial, while for others, the simplicity and user-friendliness that Alteryx provides are why they chose this software.
User interface
The main difference between Alteryx vs KNIME users in the user interface is that Alteryx has a simple menu, while KNIME users will likely deal with many superfluous windows, which consume much memory and are an issue for a slow machine.
KNIME
KNIME’s user interface consists of nodes in the repository divided into segments. Each node can be dragged and connected to other nodes onto the canvas by dragging a line from an output to an input to a data node. Also, there is an option to configure it on the spot by right-clicking the configuration settings.
Alteryx
This software has an organized UI with a menu at the top of the tool dashboard. The nodes have various options and functionalities and are categorized into color-coded categories (In/Out, data preparation, Join, Parse, data Transform, reporting, and more). Each node has a configuration that is easy to understand and edit. The interface can be enlarged or closed by clicking on the node.
Data preparation
The ETL tools are typically used to prepare data. Considering that companies spend less than 20% for data analysis, while data preparation accounts for roughly 80% of the time spent, choosing software or tool that offers simplicity and functionality is critical. Organizations collect a large amount of data daily from various sources, including CSV files, databases, and cloud sources, in both KNIME and Alteryx.
KNIME
By double-clicking on a node, users get access to various configuration options, including a file reader, the preview of the contents in the file, and opening a CSV file. Additionally, there is an option to alter the contents, including file formats and other options. One of the main advantages of KNIME is the option to filter for rows and columns, which is especially important for businesses with databases or spreadsheets with many keys. The user interface for data preparation is a bit complex for new users, which might be time-consuming when inputting or selecting a data type.
Alteryx
The panels in Alteryx allow visual drag-and-drop and datatype selection, and once the Input/Output tool is connected to a database or a file, the user gets quick visualization that includes complete information about each data point. The data cleansing tool is straightforward and user-friendly. Moreover, with the drop-down menu for selecting data types, the user can easily change the data type.
Data blending
Both KNIME and Alteryx offer powerful features for data combining, data cleaning, and data preparation process. Still, there are a few major differences:
KNIME
The Join button offers a useful function for merging and data blending from different databases on typical shared IDs that is simple and easy to learn. Also, there is an option to filter data from each data blending set and decide whether or not to include it in the final destination data blending database. Additionally, the data blending tool is super reliable when dealing with large amounts of data.
Alteryx
The Alteryx Analytic tool is less user-friendly. The Connect function allows the user to blend different databases by choosing a basic identifier and linking together various databases. However, the process is slower. The Joint tool enables users to construct SQL queries without any coding. Still, the main drawback is that Alteryx is less reliable when dealing with large amounts of data.
Data Analysis
Data scientists prefer to program their own modules, and Python is a powerful tool for this procedure. Writing custom programs to determine model behavior is feasible for perfectionists. Yet, without the knowledge of coding and computer skills, there is an extensive need for easily operated tools. And reality dictates that tools are likely to be subsequently used for advanced analytics.
KNIME
The exceptionally broad range of data analytical tools provided by KNIME makes this open-source data analytics and reporting tool a potent instrument for hundreds of developers—the wide range of plugins and adapters enables users to utilize many pre-existing functionalities. In addition, KNIME includes pre-existing ML (machine learning) style tools to allow the creation of new predictive models from current models. Another helpful feature is that KNIME can handle a variety of regressions and can, support the construction of decision trees and participate in model evaluation.
Alteryx
This software has several essential data analysis tools, such as data investigation tools, Pearson, and Spearman correlation. A few other useful features are a built-in scoring model for predicting values or dataset classes and simulation sampling. Still, if the user needs substantial modeling and testing, it is advisable to use external tools since Alteryx does not provide a wide range of analytical capabilities.
Graphing/reporting
Data science tools are often used to compare large amounts of data collected from different geolocations. As the need arises, organizations opting for ETL tools with the ability to produce a less ambiguous graphical report is very important.
KNIME
As mentioned, KNIME is an open-source and data science tool, meaning a broad variety of charts and graphs can be generated, which are extremely easy to use and create. Although it is not the primary goal for ETL tools to produce visualizations, many organizations consider it a crucial aspect of data studies. The only disadvantage is that combining these into a dashboard takes time and effort. Additionally, KNIME makes it simple to connect to BIRT (business intelligence reporting tool) and Tableau (visualization software).
Alteryx
This software offers a wide range of graphics but isn’t built for a specific purpose, meaning the visualization is not very good.
Output
After finishing the data extraction and transmission, the next step is to load or output the data into a standardized format. There are a few significant differences between these ETL tools:
KNIME
The KNIME tool offers several options for data export, including a standard CSV file and BIRT report similar to Tableau hyper file. Additionally, users can export data into various databases and formats ranging from MS SQL Server, MYSQL, PostgreSQL, and many more.
Alteryx
Alteryx’s “output data tool” enables users to link a broad range of data sources and file formats. Outputting tasks is easy and includes standard CSV flat file-like options for SQL and non-related databases and cloud-based data formats.
Choosing between KNIME and Alteryx
KNIME and Alteryx are powerful data science tools with a wide array of functions that serve individual users and businesses that handle vast amounts of data. Choosing the best tool depends on the operations the users will perform and the volume of data the organization needs. Both software tools have slightly different approaches to the ETL process.
On the one side, KNIME is a complex tool that requires solid technical background in data science and analytics but provides an outstanding ability to work with large data volumes. It is entirely modular, making it ideal for users willing to focus on developing their data science tool capabilities.
Conversely, Alteryx is a more approachable analytical tool that offers simplicity and requires fewer data input preparation. It is user-friendly and effectively handles tasks necessary for data preparation.
FAQs
What is ETL?
ETL (Extract, Transform, Load) is an integration data process used to compute and analyze data from multiple data sources and incorporate it into a single and consistent data store in a data warehouse or other target system.
What is KNIME?
KNIME is a free-to-use, open-source data analytics, and reporting tool. This Business intelligence tool has a modular data pipelining and efficiently integrates multiple data mining and machine learning components and performs data reporting and analytic operations.
What is Alteryx?
Alteryx is a data editing, analysis, and output software solution for organizations that need to handle large amounts of data. Users can prep, blend, analyze, and interpret data with easy end-to-end automation of data engineering, analytics, reporting, machine learning, and data science processes.
Is KNIME better than Alteryx?
KNIME and Alteryx offer many common features regarding data science tools. However, their differences make it easier for organizations to determine which solution suits their needs for specific use cases. For some, the customization and reliability provided by KNIME are crucial, while for others, the simplicity and user-friendliness that Alteryx provides are why they chose this software.