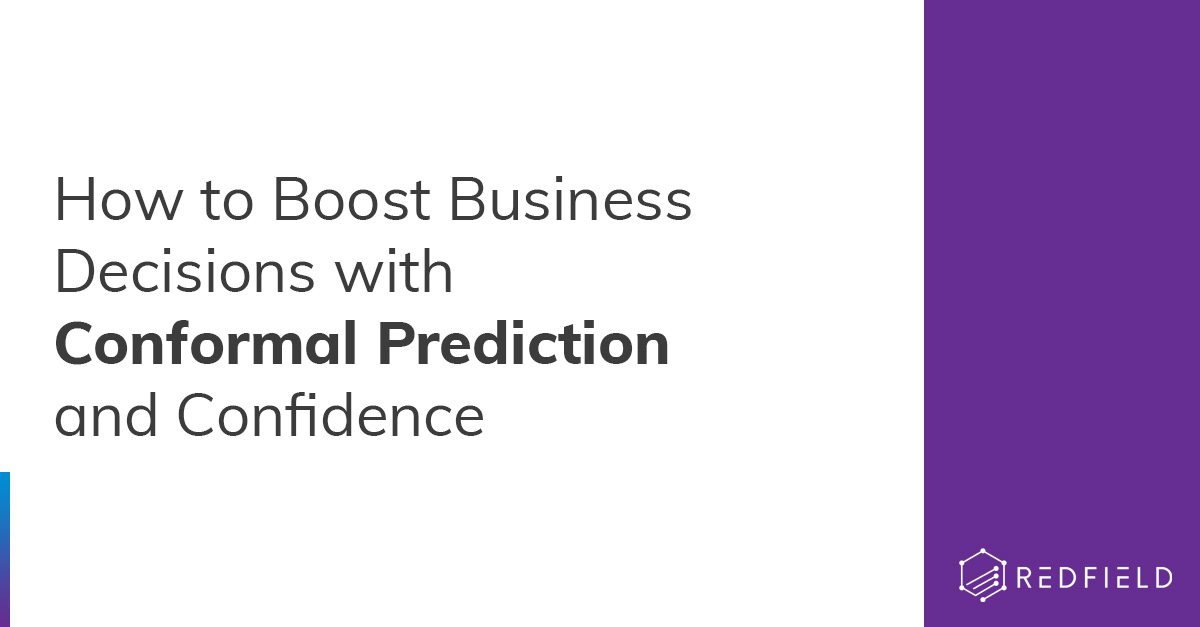
In today’s fast-paced business landscape, making accurate predictions is crucial for success. However, traditional prediction models often fail to provide a measure of uncertainty, leaving decision-makers in the dark about potential risks. Enter conformal prediction, a powerful framework that combines prediction and confidence levels, enabling businesses to make informed choices based on a more comprehensive understanding of uncertainty. In this article, we’ll explore conformal prediction and illustrate its practical value through a simple and engaging use case.
Understanding Conformal Prediction
Conformal prediction is a machine learning framework that goes beyond traditional prediction models by providing a measure of confidence for each prediction made. It acknowledges the inherent uncertainty in prediction tasks and provides a valuable tool for decision-making in a wide range of business scenarios.
Conformal Prediction: Example
Imagine you’re a sales manager at a retail company, and you want to forecast the sales volume for a new product in the upcoming quarter. Traditional prediction models would provide a single point estimate, say 10,000 units. However, this information alone doesn’t convey the uncertainty surrounding the prediction. Conformal prediction, on the other hand, provides a confidence interval, such as 10,000 units ± 500 units with a 95% confidence level. This interval determines the level of uncertainty and helps you to make more informed decisions. These decisions are based on the potential risks that might be associated with the forecast.
A Simple Use Case: Inventory Management
Let’s delve into a practical use case where conformal prediction can significantly impact business decisions. Consider a company that manufactures and sells electronic gadgets. Effective inventory management is important if you want to avoid stock outs or excess inventory, both of which can harm the company.
To optimize inventory levels, the company needs accurate sales forecasts along with a measure of confidence. By leveraging conformal prediction, the company can predict future sales volumes for each product with associated confidence intervals. Let’s say they use historical sales data, product features, and external factors (e.g., economic indicators) as input for the prediction model.
Suppose the conformal prediction model forecasts that 5,000 units of a particular gadget will be sold in the next month, with a 90% confidence interval of ± 300 units. Armed with this information, the company can make data-driven decisions:
1. Ordering Inventory
The company can adjust the reorder point and order quantity based on the forecasted demand and the associated confidence interval. For instance, they might choose to order 5,300 units to ensure they don’t run out of stock during the month.
2. Marketing and Promotions
The confidence interval can help plan marketing campaigns and promotional activities. If the lower bound of the interval indicates strong demand, the company can invest more in advertising and promotions to maximize sales. Conversely, if the upper bound suggests weaker demand, they can focus on cost-saving initiatives.
3. Supply Chain Management
The company can work closely with suppliers and logistics partners to align production and delivery schedules based on the forecasted demand and confidence intervals. This proactive approach helps prevent unnecessary inventory buildup or supply shortages.
How Conformal Prediction Is Used In Quality Control
A steel plant can utilize conformal prediction for quality control by training models with historical data to predict acceptable quality ranges. This enables proactive identification of potential defects and minimizes false positives. By incorporating uncertainty estimates, the plant can make informed decisions, reduce wastage, and enhance overall product quality, boosting customer satisfaction.
A technical specialist in a steel manufacturing plant can leverage conformal prediction for quality control in the following manner:
- Firstly, the specialist collects historical data on various production parameters, such as temperature, pressure, and composition, along with corresponding quality outcomes.
- Using this data, a conformal predictor is trained to estimate the confidence intervals for future quality measurements.
- When a new steel batch is produced, the specialist can input the real-time process parameters into the conformal predictor, which will generate reliable prediction intervals for the expected quality.
- By comparing the actual quality measurement with the prediction intervals, the specialist can quickly identify potential defects or deviations, enabling proactive interventions and ensuring consistent high-quality steel production.
Conclusion
Conformal prediction is a valuable framework that empowers business decision-makers by providing predictions accompanied by confidence intervals. This approach enhances decision-making processes by quantifying uncertainty, leading to more informed actions. By leveraging conformal prediction, companies can optimize inventory management, fine-tune marketing strategies, and streamline supply chain operations. Embracing this powerful tool can give businesses a competitive edge in today’s unpredictable marketplace, enabling them to make smarter and more confident decisions.