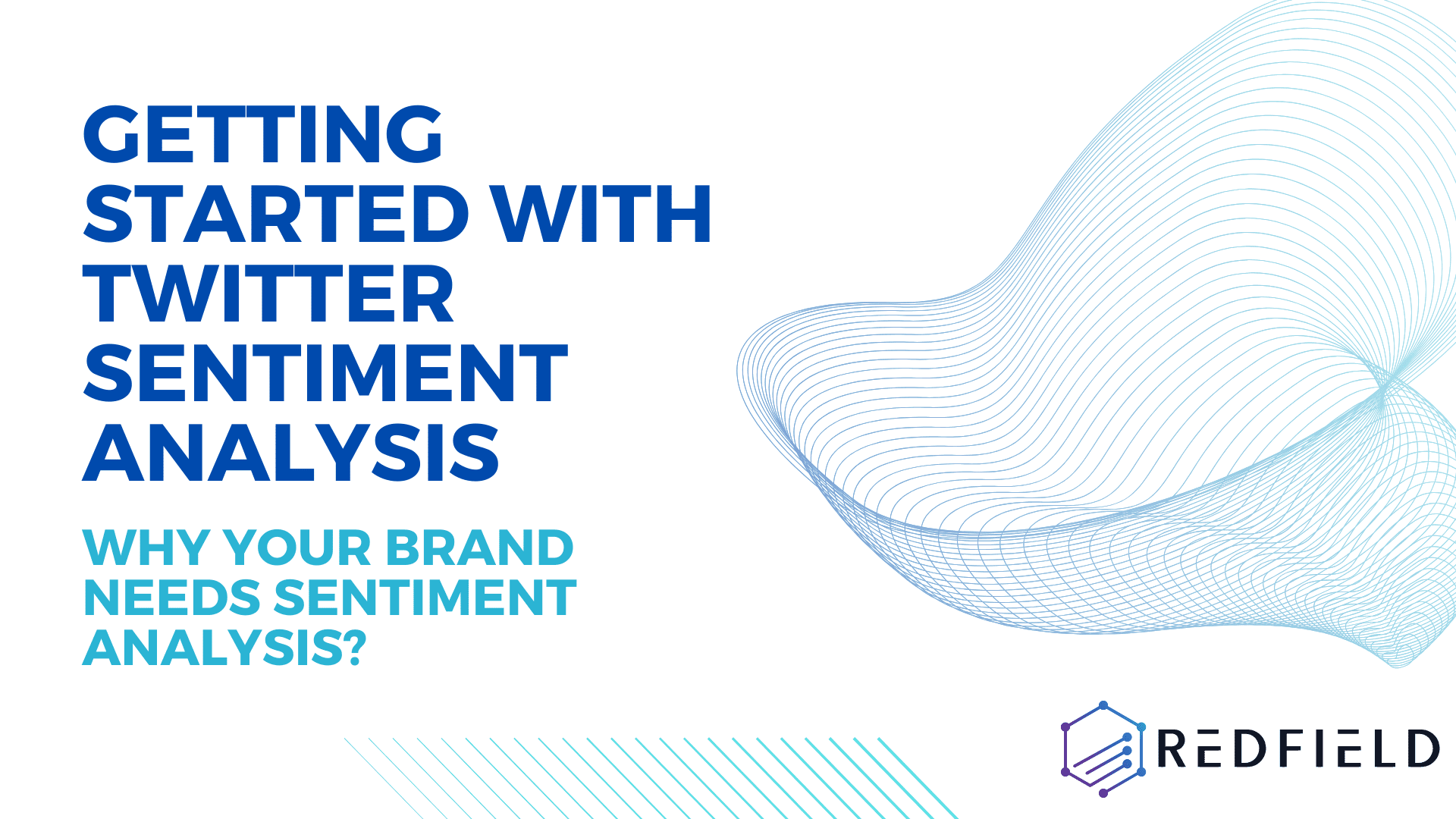
With the advancement of web technology and its growth, a massive volume of data is presented to internet users, and big data is generated daily. The Internet has become a platform for online learning, exchanging opinions, and sharing ideas. Social networks like Twitter, Instagram, Facebook, Google+, YouTube, and TikTok are rapidly gaining popularity as they allow people to express their views about topics and have discussions with different communities worldwide. Social media platforms, and Twitter in particular, are a goldmine for harvesting honest feedback and true opinions, and consequently, there has been much work in the field of Twitter sentiment analysis.
According to Statista, the social network X/Twitter is top-rated in the United States, and as of January 2023, the microblogging service had an audience reach of 95.4 million users, and as of the second quarter of 2021, Twitter had 206 million monetizable daily active users worldwide. Indeed, Twitter is a treasure trove for businesses. With such a vast potential market for gathering opinions about various products and services, companies are confident to invest heavily in better understanding behavioral patterns and people’s views to tailor their offerings and build a loyal customer base.
Twitter is a space where users share their thoughts and opinions, connect with others, communicate, and contribute to specific topics using short, 140-character posts, known as tweets. The interaction is performed by using likes, comments, and repost buttons on the texts, pictures, and videos that users share. The more people contribute to social media, the analysis of information available online will better reflect people’s perceptions, behavior, and interests about specific topics. Hence, using Twitter data for sentiment analysis has brought more attention to Natural Languages Processing (NLP) and Artificial Intelligence (AI) technologies related to text analysis.
Table of Contents
What Is Sentiment Analysis?
Sentiment refers to the positive or negative view or opinion held or expressed in text. Sentiment analysis is a field of study that uses computational methods to analyze, process, and reveal people’s feelings, sentiments, and emotions hidden behind text or interaction. This type of text analysis effectively evaluates written or spoken language and determines if the expression is favorable (positive), unfavorable (negative), or neutral and to what degree.
If you have ever left an online review, commented about a brand or product online, or answered a market research survey about certain products and services, there’s a chance that your feedback has been through sentiment analysis.
With a combination of methods and techniques like using machine learning algorithms (ML), natural language processing (NLP), data mining, and artificial intelligence (AI) techniques to mine, sentiment analysis extracts and categorizes users’ opinions on an organization, product, service, person, event, or idea for various sentiments. The data provides a helpful indication of how customers felt about their experience, and businesses leverage the information to make well-informed decisions.
Sentiment analysis is part of a greater umbrella of text mining, also known as text analysis. The contextual mining of text identifies and extracts subjective information from the source material and helps businesses understand their brand’s social sentiment in general or the products and services they offer.
While monitoring online conversations, text analysis extracts meanings from different text sources, such as surveys, reviews, public opinion mining social media and social listening, blogs, and articles on the Web. A score is then assigned to each clause based on the sentiment expressed in the text. For example, -1 represents negative sentiments and +1 positive sentiments. The sentiment analysis tools can consistently and accurately handle large volumes of data and reveal how customers feel about topics, ranging from the businesses’ products, customer services, ad campaigns, or even competitors in the area.
What Is Sentiment Analysis Used For?
Accurate sentiment analysis can be challenging to conduct. Is it worth investing funds in AI-powered tools to categorize natural language feedback rather than employ human brains?
Generally speaking, it’s a question of scale. Sentiment analysis is performed when companies have a large volume of text-based information and need to extract the information from that extensive text data set. For example, after releasing a trailer for a movie that has received a considerable volume of comments on Twitter, the marketing team of that motion picture studio needs to analyze the statements.
Reading of all comments is time-consuming and expensive. Even after reading many comments, the person studying them won’t be able to get an accurate picture of how many people liked or disliked the movie unless they make a note of each comment, whether it was positive, negative, or neutral. Additionally, there is a high risk of bias coming from the individuals or groups reading the comments, and certain data might be wrongly interpreted based on changes in their judgment, mood, energy levels, and other variations.
On the other hand, sentiment analysis provides a comprehensive, consistent overall finding with simple clicks and commands. After providing the analysis, companies determine how to put the sentiment into action.
How Does Sentiment Analysis Work?
Sentiment analysis uses machine learning models to conduct text analysis of human language. The metrics used in the sentiment analysis model are designed to detect whether the overall sentiment of a piece of text is positive, negative, or neutral. Generally, the most perform sentiment analysis follows several steps:
Collect data
The first step is to identify and collect data. Often, this process employs web scraping techniques.
Clean the data
The collected data is then processed and cleaned to remove noise and parts of speech that don’t have meaning relevant to the sentiment. Examples of such data are phrases such as contractions (I’m), words that have little information (is), articles (the), punctuation, URLs, special characters, and capital letters.
Extract features
A machine learning algorithm is trained to automatically extract text features and identify negative and positive sentiments.
Pick an ML model
By choosing between a rule-based (uses predefined and lexicon-based rules), automatic (ML and deep learning), or hybrid ML model (combination of both approaches), the sentiment analysis tool scores the text and assigns sentiment scores to pieces of text.
Sentiment classification
After selecting the model and employing it to analyze a piece of text, the model assigns a sentiment score to the text, including positive, negative, or neutral.
Sentiment Analysis Use Cases
With the explosive rise of Internet use, news articles, social media, and digital communications, an enormous amount of user-generated data is being created consistently. People publish comments and give reviews to express their feelings based on common objects of interest. Sentiment analysis helps companies track how users perceive their brands and products at crucial moments and over a specific period.
Sentiment analysis is also used in market research, marketing analysis, PR, stock analysis and financial trading, medicine, reputation management, customer experience, product design, and many more fields. For example, there are a few scenarios where sentiment analysis can save time and money and add great value:
- Social media listening – the practice of monitoring social media channels can be performed daily or around a specific event, such as a product launch or Black Friday sales.
- Analyzing survey responses for large-scale research programs for companies monitor various industries.
- Processing employee feedback in large corporations.
- Identifying dissatisfied customers helps businesses to offer closed-loop follow-up and increase customer satisfaction.
- Analysis of clustered sentiment trends in specific groups or regions.
- Competitor research – analysis of competitors in terms of product price and range, launch of new products, etc.
What Is Twitter Sentiment Analysis?
A Twitter sentiment analysis identifies negative, positive, or neutral emotions within the text of tweets. In the process of analyzing tweets, the text analysis employs natural language processing (NLP) and machine learning (ML) to identify and extract the subjective information contained in original data, thus providing a company with valuable insights in terms of the social sentiment of the brand, products, and services.
The stockpile of information helps organizations to analyze users’ reactions, attitudes, emotions, and opinions and is particularly beneficial in forecasting product sales, trends in the stock market, and results of political elections. With over 500 million monthly active users and exceptional significance in the perception of people’s thoughts and attitudes, Twitter is one of the most popular micro-blogging services and has attracted a great deal of attention from companies worldwide.
The business’s classification and analysis of customer conversations interlinked with a certain brand is crucial. The characteristics are a tool for analyzing conversations concerning the brand and brand reputation. Two aspects that are pivotal for companies are:
- The most relevant characteristics of the products and services that customers like or dislike
- The customer behavior and intentions with respect to the brand characteristics
Why Is Twitter Sentiment Analysis Important?
The company’s qualitative data gets its sense with sentiment analysis with information gathered through different platforms, such as Twitter.
Voice of Customer (VOC) Programs
The feedback gathered from Twitter data helps companies to understand customers’ feelings, preferences, likings, and concerns about a brand. The analysis of Twitter sentiment regarding specific brands gives insights and helps companies with risk prevention and strategy development for better-fixing issues concerning the brand’s products and services.
Evaluating how customers feel about the brand and their opinions about the products and services assist in distinguishing and addressing the issues before they arise, which is why the Voice of Customer (VoC) programs are crucial for improving customer experience.
Customer Service Experience and Customer Success
Businesses depend on their customers, and extraordinary client involvement can make or break a company. Estimating the user’s opinions and assumption examination can give interesting insights that help companies create better management strategies and thus increase productivity, decrease handling times, and improve overall customer experience, leading to customer success.
Did you know that the average response time for businesses on Twitter is about one day and seven hours? Even more interesting is that approximately 64% of customers want a reply within an hour of posting, and brands strive to do that. For businesses, it is the primary goal to track high-priority issues that are critical and require an immediate response, and Twitter sentiment analysis is a creative technique that enables that.
Undoubtedly, Twitter has become one of the prominent platforms for expressing appreciation and resentment, meaning brands must be ultra-proactive in addressing queries, positive reviews, or frustrations and bridging the gap between brands and customers. Additionally, employees can be the brand voice that can strengthen the brand-to-customer relationship with a personal touch.
Product Experience
Everything related to a specific brand is about its products and services and the revenue it generates quarterly or annually. Twitter sentiment analysis can identify how clients feel about the highlights and benefits of the brand’s products and services. Furthermore, this can reveal areas of interest and opportunities that may not be mindful or apparent to target audience.
Through Twitter conversation, companies can gather twitter data to pinpoint online item audits for criticism of specific item categories over competitors in the showcase. With estimation examination, managers can uncover subjects that users consider good or bad and find openings or joint issues.
Brand Sentiment Analysis
The sentiments adjoining the brand are one of the predominant factors in good customer experience. Based on the brand sentiment, if positive tweets are dominant, sales can increase or decrease based on negative sentiments. The sentiment analysis results also reflect brand loyalty, where positive sentiments result in good reviews and recommendations, and on the other hand, negative mentions increase customer churn rates.
Twitter sentiment analysis provides brands with tools to gather tweets and monitor negative mentions about how their customers feel about their business. Studying the Twitter conversation and analyzing the relevant tweets is advisable for companies to analyze the brand’s reputation. Companies need to track how their brand is performing, and analyze tweets about product names and features, and pay attention to competitor mentions to understand the big picture.
Real-Time Supervision
Brand monitoring is a complex process that maintains brand reputation and the online reputation of a particular brand. From customer feedback analysis to monitoring Twitter mentions about the brand and its products and services, new launches, ongoing campaigns, sales, and much more. The machine learning models perform sentiment analysis and enable businesses to perform end-to-end SWOT analysis, leading to better decision-making and prompt improvements based on customer concerns and recommendations.
Market Research
Twitter sentiment analysis can offer assistance to companies in distinguishing new trends, analyzing competitors, and testing emerging markets. For instance, with sentiment analysis, businesses can analyze the sentiment analysis results and scores of competitor reviews and evaluate what clients like or dislike about the competitor’s product features.
Moreover, Twitter sentiment can be the key instrument in determining customers’ pain points and notable discernments. It can track keywords, and by analyzing tweets, the tool can compute the customers’ perceptions.
The multitude of topics discussed on Twitter and the freely expressed thoughts and convictions about plenty of subjects help businesses to identify customers’ persona, behavior, likes, and dislikes based on current trends and interests.
Political campaigns and public actions
The track record of news and debates on political views is an outstanding package used for Twitter analysis. In the scenario for political campaigns and public actions, monitoring the inconsistencies in Twitter sentiment can detect potential issues and areas that require public action.
Twitter sentiment analysis can help gauge election results way before the elections have happened. Research shows that Twitter sentiment strongly paved the way for Donald Trump’s win in the 2016 USA Presidency. For example, with Twitter sentiment analysis, MonkeyLearn compared the polarity of tweets related to Trump and Clinton during the 2016 Presidential elections. According to the analysis, there were positive, negative, and neutral sentiments across days until the day of the election, and the two graphs plainly show what led to Trump’s final win.
With the users’ honesty, Twitter is an excellent place to discover the blueprint of public opinion about any topic. Still, with politics being a sensitive topic, such campaigns could be outrageous and are crucial to predict public actions and avoid dangerous circumstances.
How to Do Twitter Sentiment Analysis?
Twitter is a massive platform on which people interact via tweets about a wide range of topics and with different communities. Companies can significantly benefit from Twitter data by collecting opinions related to them, and the steps to perform Twitter sentiment analysis are:
Extract and Collect Twitter Data
Data extraction is the first and foremost crucial stage. All of the subsequent phases of sentiment analysis depend entirely on it. Therefore, ensuring that Twitter data encompasses exact observations that the brand aims to analyze is substantial.
To gather information from Twitter, companies should take under consideration two factors:
– Current (latest) tweets are helpful for tracking keywords, hashtags, or topics that appear in real-time
– Historical tweets are valuable for comparison of sentiments over different periods
Prepare Twitter Data
Generally, tweets are unstructured data and extensively require preprocessing before using the information as training data for the Twitter sentiment analysis model. Needless to say, the better the quality of content from Twitter data, the better the results will be.
As mentioned above, preprocessing of the data set includes:
- Cleaning of noisy data
- Deleting duplicates or meaningless tweets (shorter than three characters)
- Formatting improvements and sequences
- Preparing data to perform testing of the model
In this step, removing irrelevant information, such as emojis, extra whitespace, off-topic references, and discussions, is essential. The goal is to facilitate high-quality, clean, and good data and produce precise outcomes that contain accurate and valuable predictions without human error.
Build Twitter Sentiment Analysis Model
The steps in building machine learning models vary depending on the problem statement, requirements by the client, and use case. However, several model-building steps should be included in Twitter sentiment analysis:
- Create a base model type as a ‘Classifier’ to define and categorize tags for the sentiment analysis model
- For classification, create a sentiment analysis project under the model
- Import the preprocessed data as a training dataset for the model
- Train the model: Classify each tweet as positive, negative, or neutral
- Test the model performance and preciseness of the generated predictions
The tags in training data can be defined more explicitly for increased accuracy. Additionally, the preciseness will be significantly increased if the false positives and negatives are crosschecked with different test cases.
Analyze Twitter Data for Sentiment Analysis
The most straightforward stage is when qualifying Tweets can be submitted to a sentiment analysis tool. In this step, the user needs to integrate the Twitter data with the Twitter sentiment analysis model. There are pre-built Twitter APIs that simplify the integration process.
You can also check this video about how to perform Twitter sentiment analysis in Python:
Demonstrate Results Using Data Visualization Tools
Sentiment analysis generates KPI results that are best presented through graphs and charts. The data obtained from the Twitter sentiment analysis report is best illustrated with data visualization tools. With data visualization, results are presented in an easy-to-understand, interactive, and dynamic form, making the Twitter sentiment analysis uncomplicated, convenient and highly scalable method for data analysis. Additionally, with the help of different types of dashboards, such as customer service dashboards or sales dashboards, companies can track KPIs and monitor overall productivity.
The brand’s online reputation requires established significance and trustworthiness of the business’s online presence, and the analysis of Twitter sentiment is excellent for raising the reputation score online. Online reputation is one of the most critical assets since potential customers can quickly learn about any company with a few clicks and analyze the brand’s footprints over time. Users form their opinions and make purchasing decisions based on impressions, including photographs, comments, retweets, and posts on Twitter and other social media channels.
To Sum Up
The entire world is transforming quickly under the present technological innovations. Today, the Internet is a basic requirement for millions of people, and the Web is being used in every field, from random chats between friends to political studies and surveys. With the rapid increase of social network applications, people are taking the freedom to use these platforms to voice their opinions about daily issues, concerns about the future, and real-life scenarios.
Gathering and analyzing people’s reactions toward buying a product or choosing a service is vital for companies of all sizes and industries. Sentiment analysis discovers the sentiments behind opinions in texts on various subjects. Twitter sentiment analysis, in particular, is the prospective tool for measuring social studies.
On Twitter, millions of people voluntarily write their opinions on a plethora of topics related to opinion leaders, key influencers, relevant brands, and much more. Twitter is likely the best place to get a sampling of public opinion without limitations. Sentiment analysis of tweets gives a comprehensible and coherent perspective on users’ sentiments concerning products and services, market trends, and successes and failures of businesses.
What Is Sentiment Analysis?
Sentiment analysis is a field of study that uses computational methods to analyze, process, and reveal people’s feelings, sentiments, and emotions hidden behind text or interaction.
What Is Sentiment Analysis Used For?
Sentiment analysis provides a comprehensive, consistent overall finding with simple clicks and commands. After providing the analysis, companies determine how to put the sentiment into action.
What Is Twitter Sentiment Analysis?
A Twitter sentiment analysis identifies negative, positive, or neutral emotions within the text of tweets. In the process of analyzing tweets, the text analysis employs natural language processing (NLP) and machine learning (ML) to identify and extract the subjective information contained in original data, thus providing a company with valuable insights in terms of the social sentiment of the brand, products, and services.
Why Is Twitter Sentiment Analysis Important?
With Twitter sentiment analysis, companies analyze the Voice of Customers (VOC), customer service experience, customer success, and product experience. Additionally, companies perform brand sentiment analysis, real-time supervision, and market research.
How to Do Twitter Sentiment Analysis?
The first step is to extract and collect Twitter data and then prepare the data for analysis. The next step is to build a Twitter sentiment analysis model and analyze Twitter data for sentiment analysis. Ultimately, the information from the analysis is demonstrated with data visualization tools.